ABOUT
Data Scientist & Economist
I'm a Data Scientist at Google.
My research focuses on applied econometrics, empirical IO, and the intersection of data science and machine learning.
I obtained my Ph.D. in Economics from the University of California—Irvine.
SKILLS
Languages & Techniques
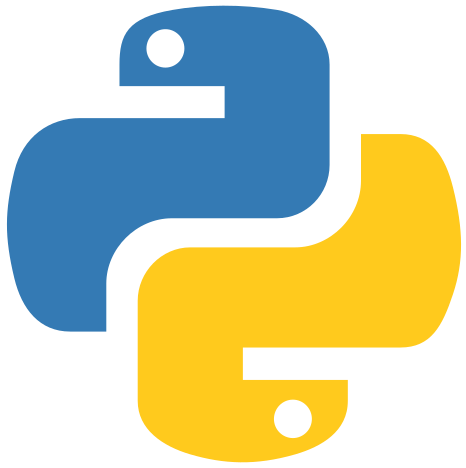
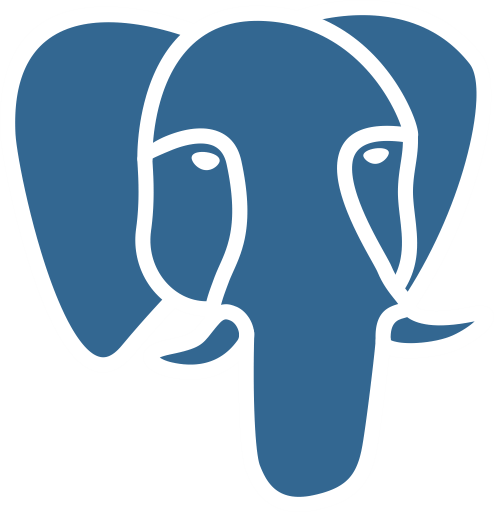
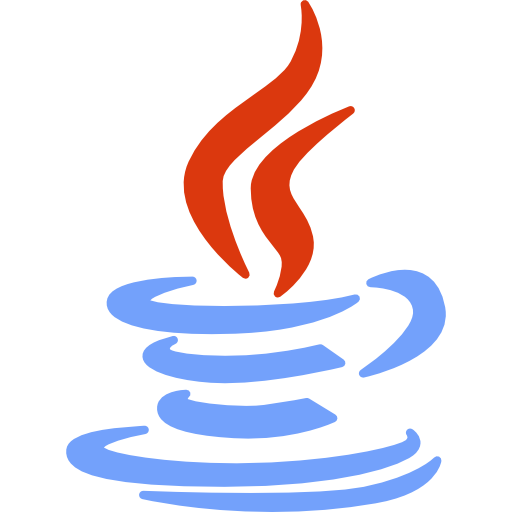
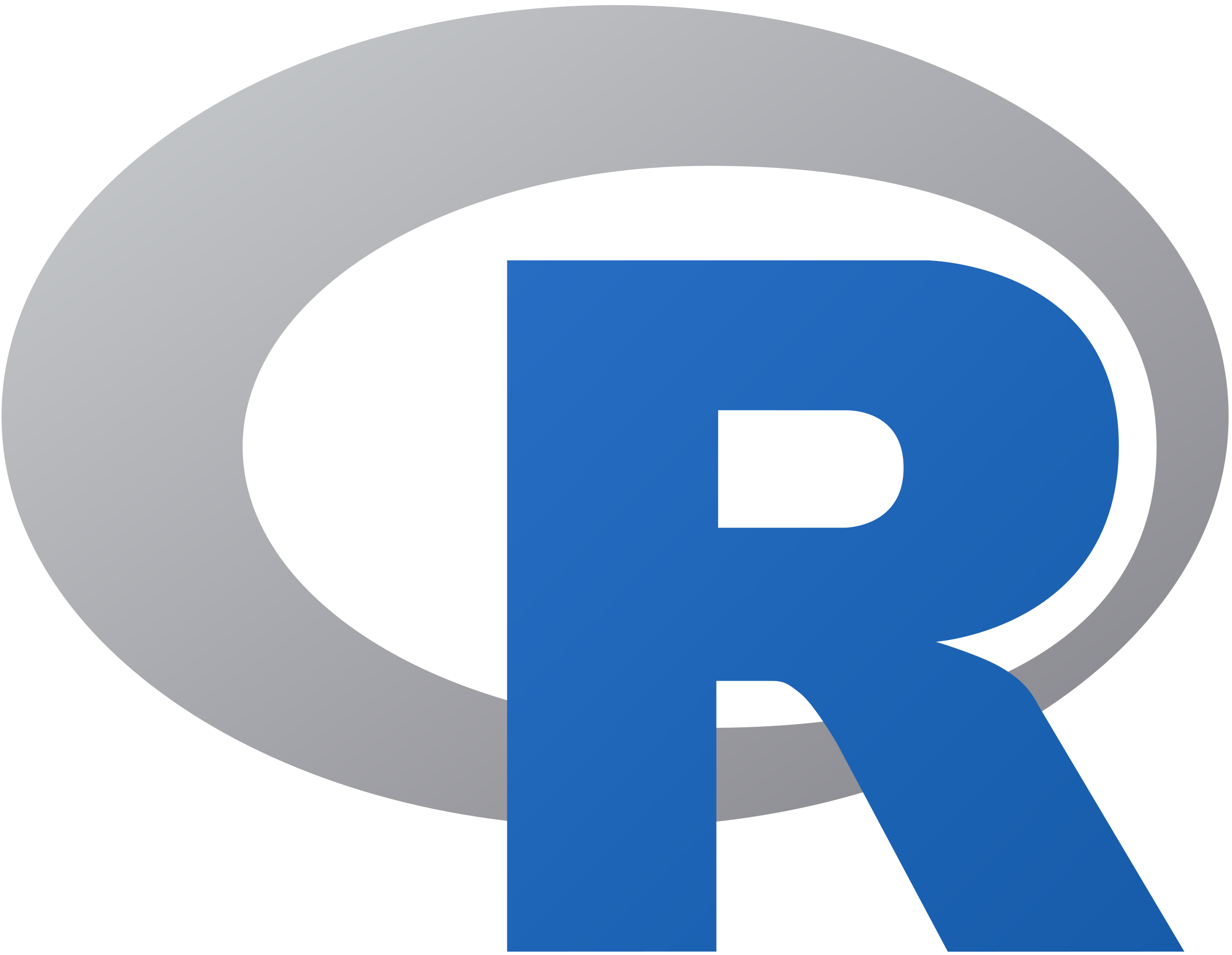
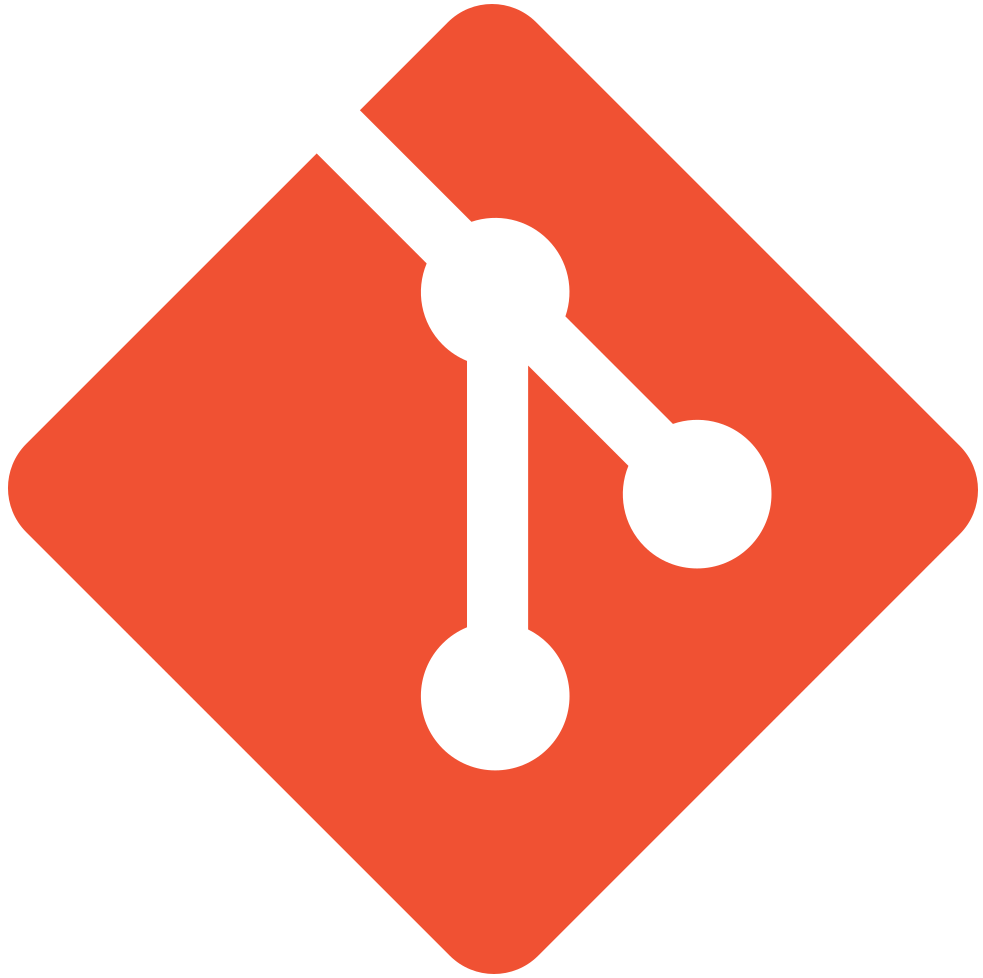
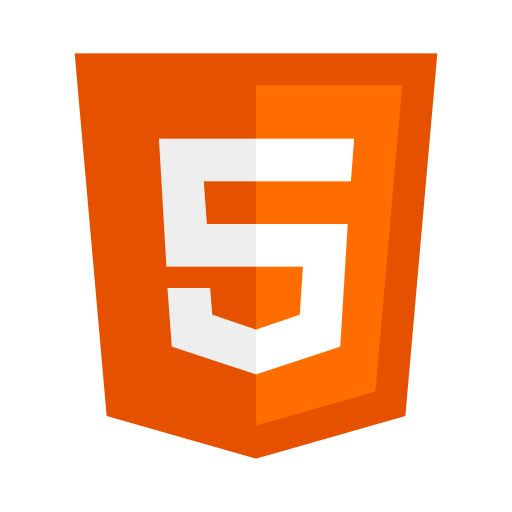
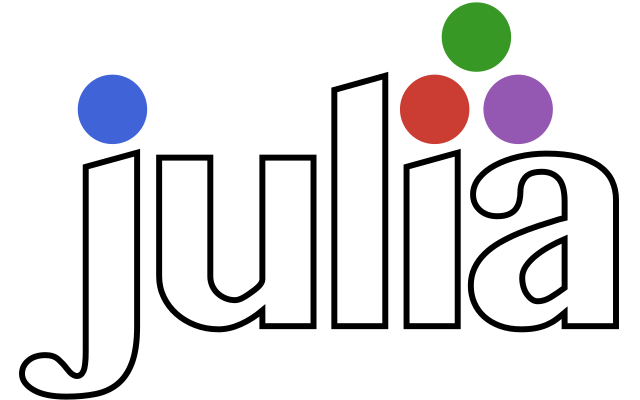
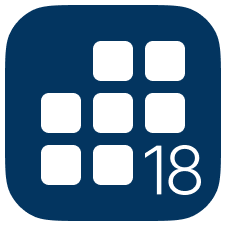
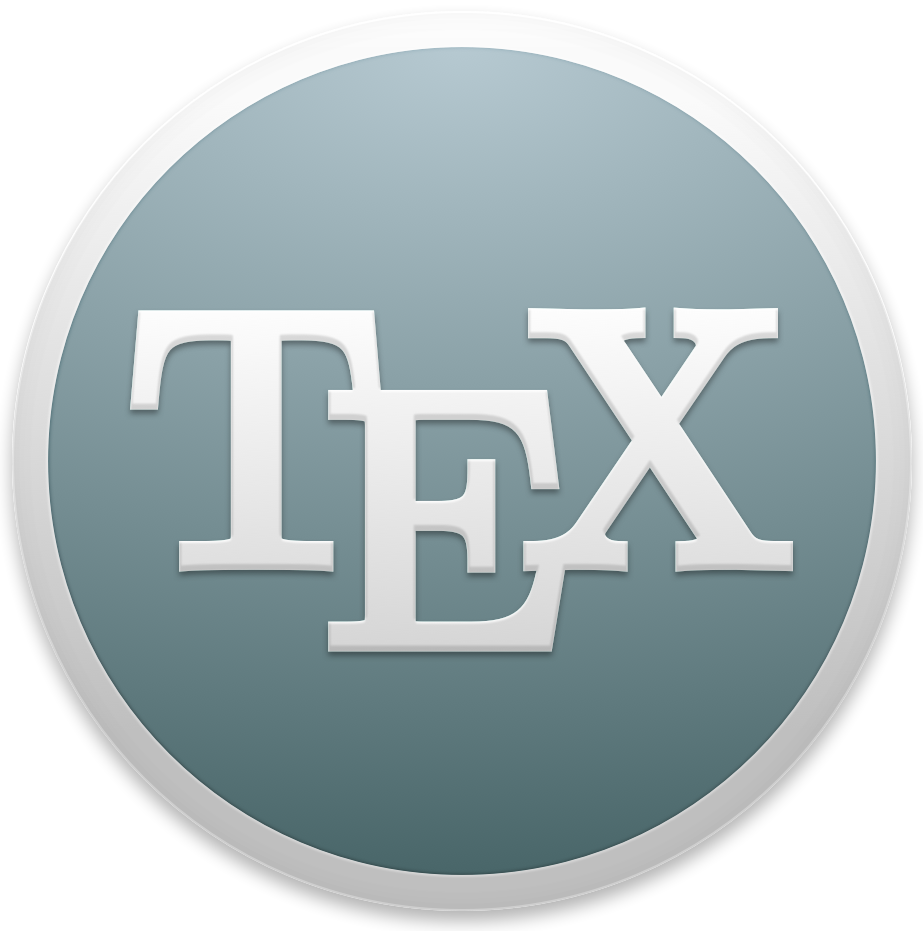
RESUME
Experience
Google2023 —
Data Scientist
Operations & Infrastructure Data Science
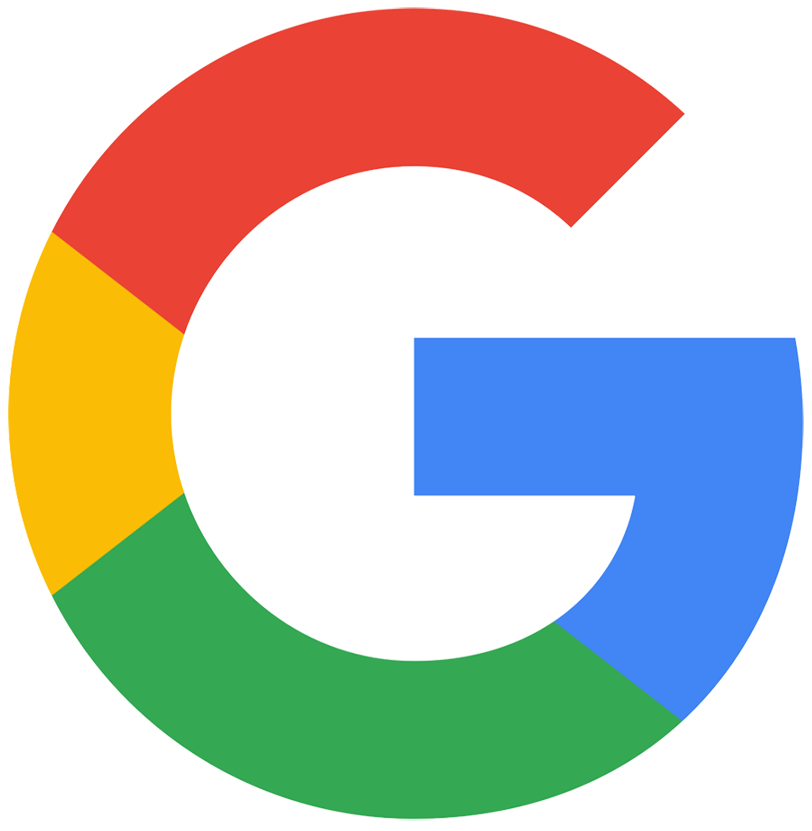
HP2023
Economist Intern
Pricing Analytics
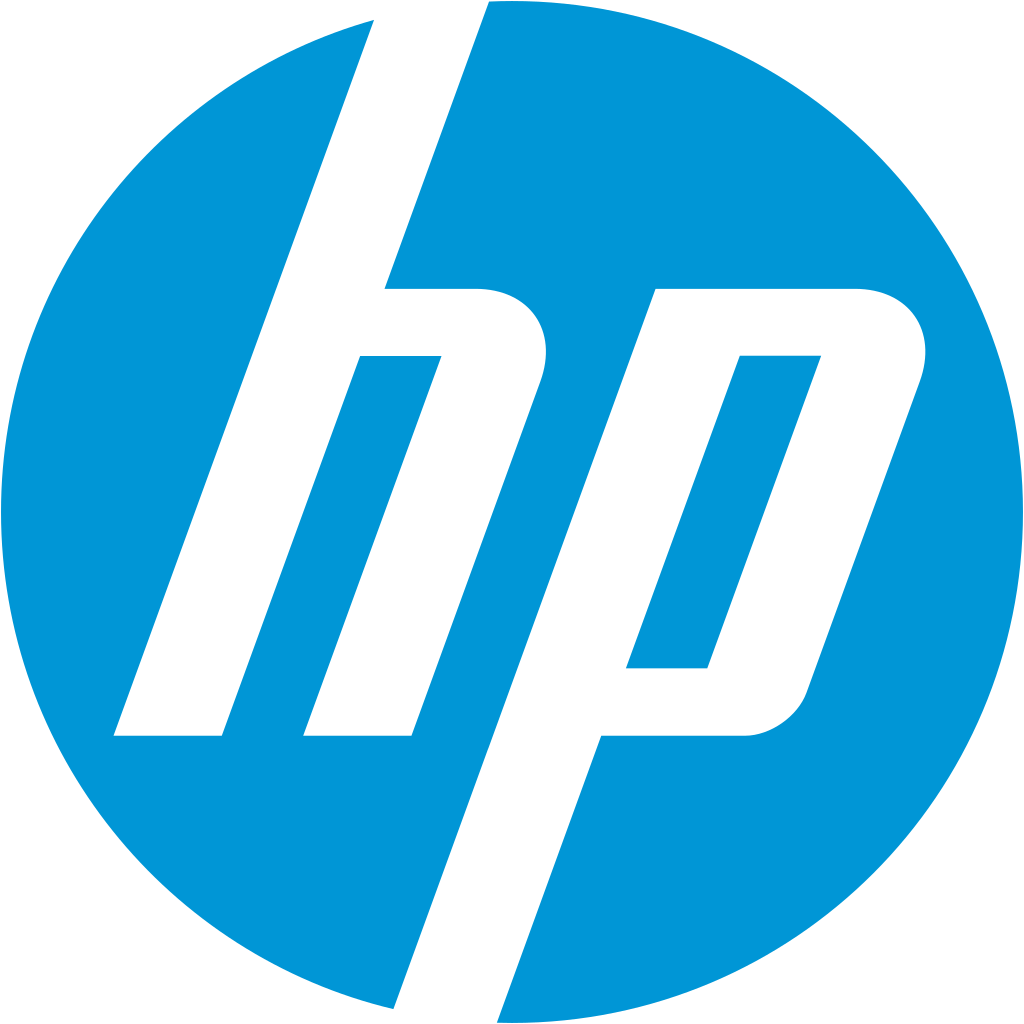
Google2022
Data Scientist Intern
Glassbox Learning
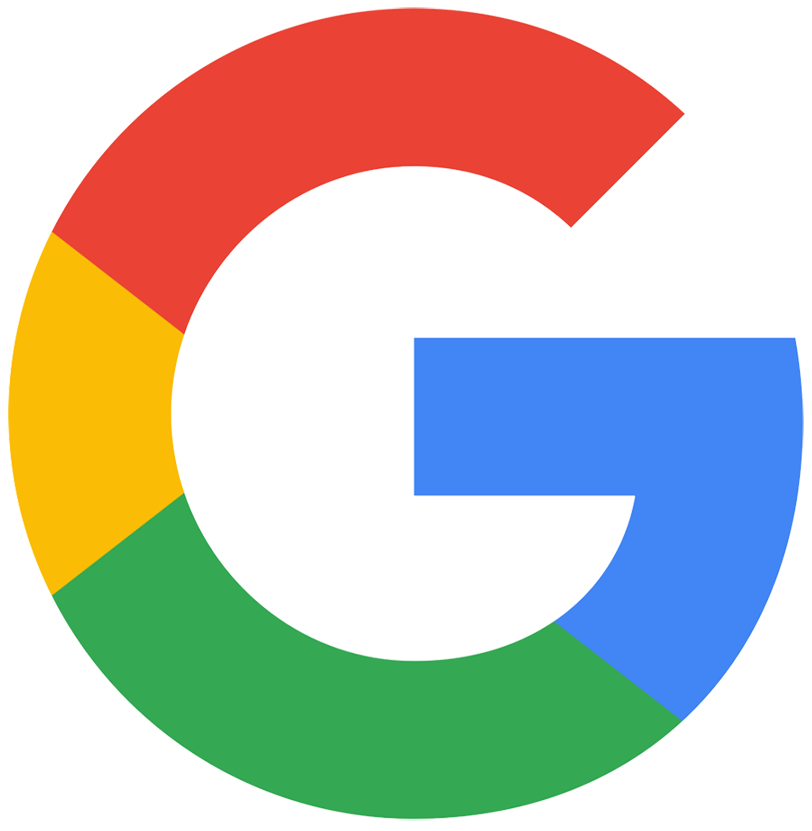
Amazon.com2021
Economist Intern
Prime Video
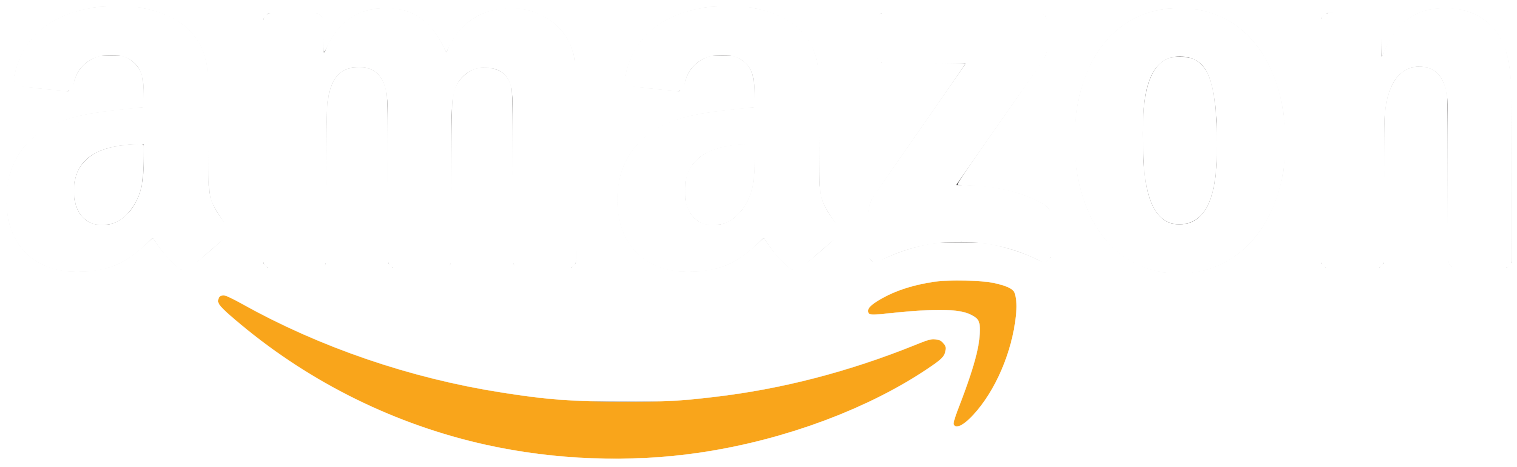
Education
Ph.D. in Economics
University of California—Irvine
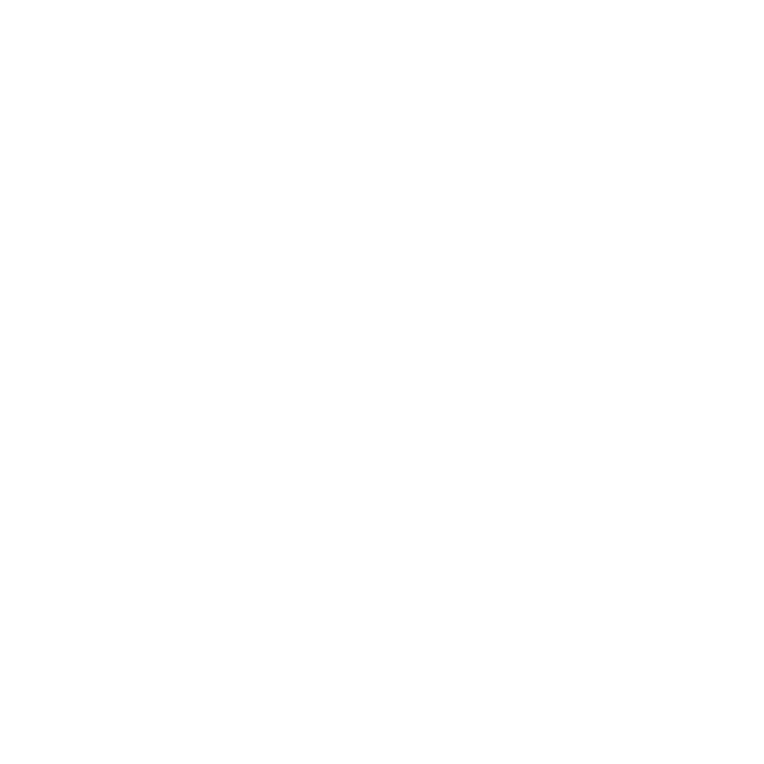
M.S. in Economics
University of Wisconsin—Madison
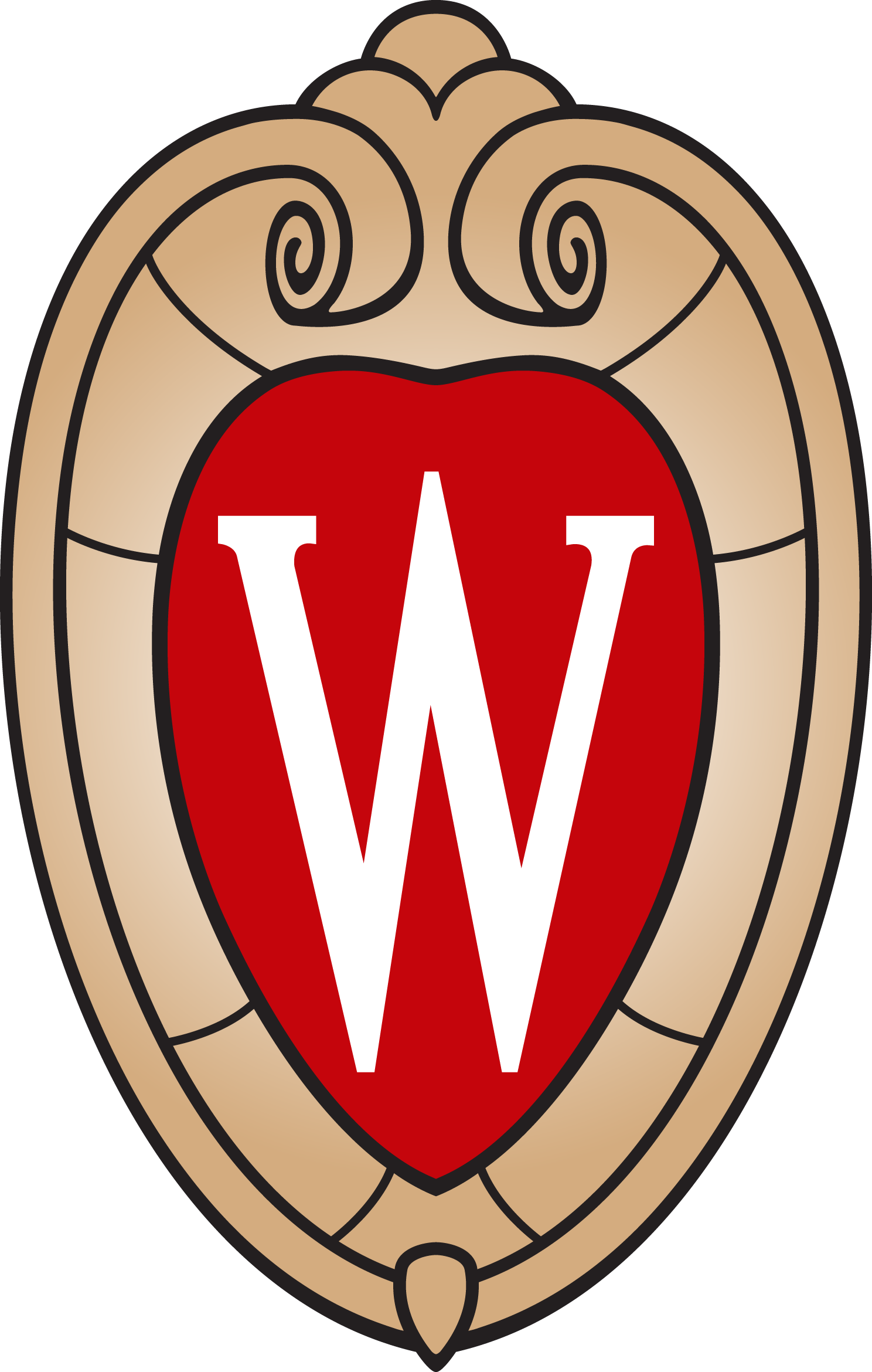
B.A. in Economics
National Chung Cheng University (CCU)
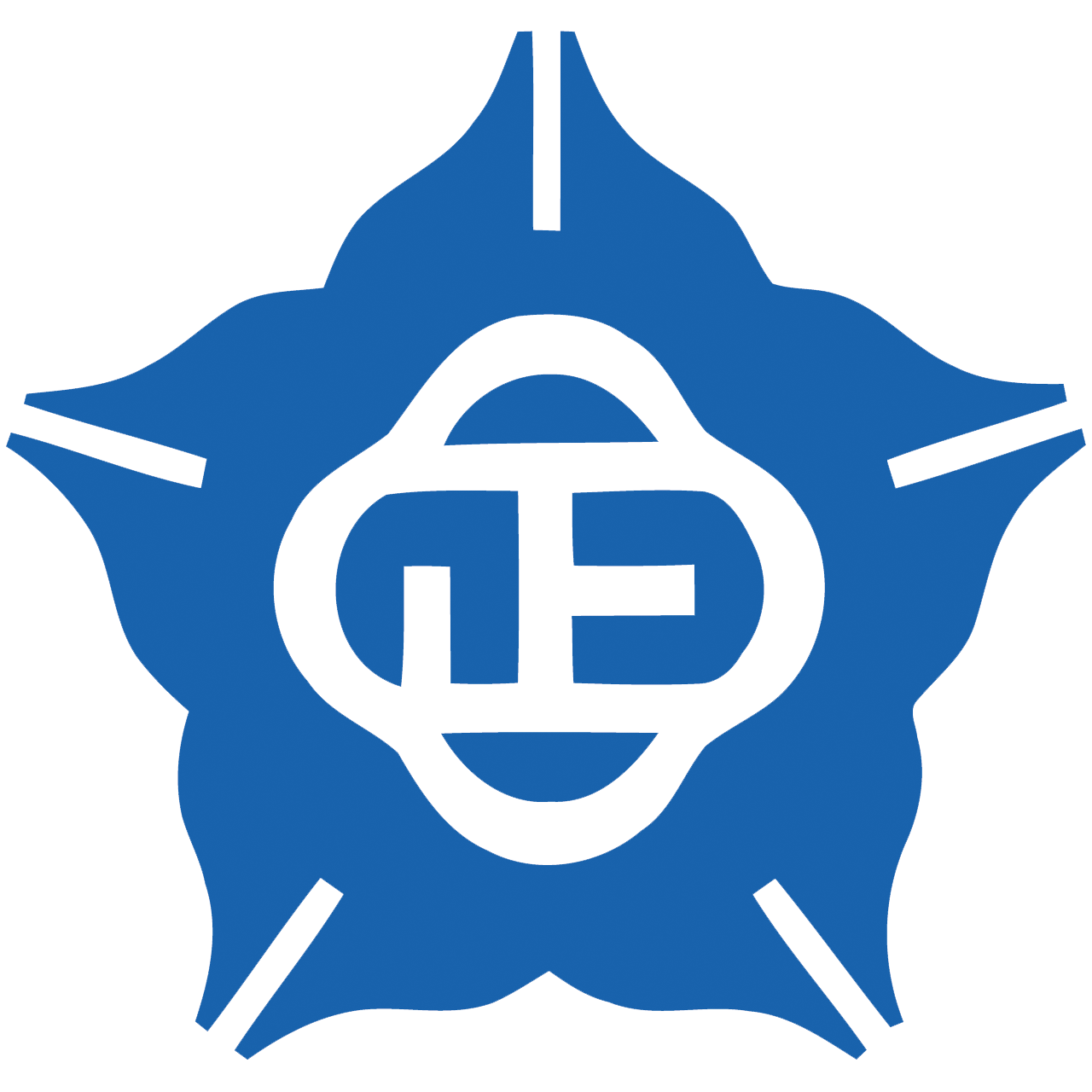
RESEARCH
Projects
Selection of prior work and visualizations.
-
Map of Alternative-credit Loan Inquiries
Vue.js
Highcharts
Map of ALT-credit Loan Inquiries (ALT Loan Lab)Please view this interactive map on desktop browsers.
-
Product Level Hierarchy Classification with Transformer-based Clustering
ML
I utilize a sentence transformer to embed product names with BERT models. The products gathered from online stores are projected into the embedding space and grouped into finer COICOP categories to calculate price indexes.
Unlike traditional NLP models, the Transformer-based model evaluates sentence tokens simultaneously. The inputs are represented by a vector of embeddings that incorporate position and attention information. The high-dimensional embeddings are reduced to ten principal components and clustered with the EM algorithm. -
Community Detection on a Social Network
Graphical Models
This project investigates various community detection techniques using network data from the Hornet social platform. The approaches compared include Mixed Membership Stochastic Blockmodels and K-means clustering on both node edges and individual demographic features. The likelihoods of different sampling methods for the stochastic model are evaluated and the network topology is visualized using Gephi.
-
Random Coefficient Logit Model with MCMC Algorithms
Metrics
Numerous techniques have been developed to solve the random coefficients logit model. Following a developed method, I modify the prior distribution assumption on the aggregate demand shocks and estimate demand by sequentially updating the market share inversion process with Gibbs and Metropolis-Hasting sampling methods. In particular, I present a practitioner's guide including details of the algorithms' implementations.
-
Image Generation with an Introspective Deep Learning Algorithm
ML
This project re-implements the introspective variational autoencoder to synthesize realistic images. IntroVAE repurposes the inference model to additionally act as a discriminator, enabling the model to self-estimate differences between generated and real images in an adversarial manner.
We replicate and deliver comparable image quality to those presented in the research, and confirm the advantages of this model over standard VAEs and GANs.
LINKS
Blog / Notes / Groups
-
Blog
-
Notes and Templates
-
Groups
-
Links
Handout
Teaching
-
ECON 20A/23: Basic Economics I
-
ECON 105A: Intermediate Quantitative Economics I
2020 Fall
-
ECON 13: Global Economy
-
ECON 142CW: Industrial Organization III
2020 Spring
-
ECON 20B: Basic Economics II
-
Game Theory (I)
CCU, 2013 Fall
-
ECON 134A: Corporate Finance
2020 Summer I
-
ECON 100C: Intermediate Economics III
CONTACT
Let's get in touch
Email: howard@hsu.xyz
Website: haochehsu.com
Location: Bay Area
Copyright © Hao-Che Hsu. All rights reserved.